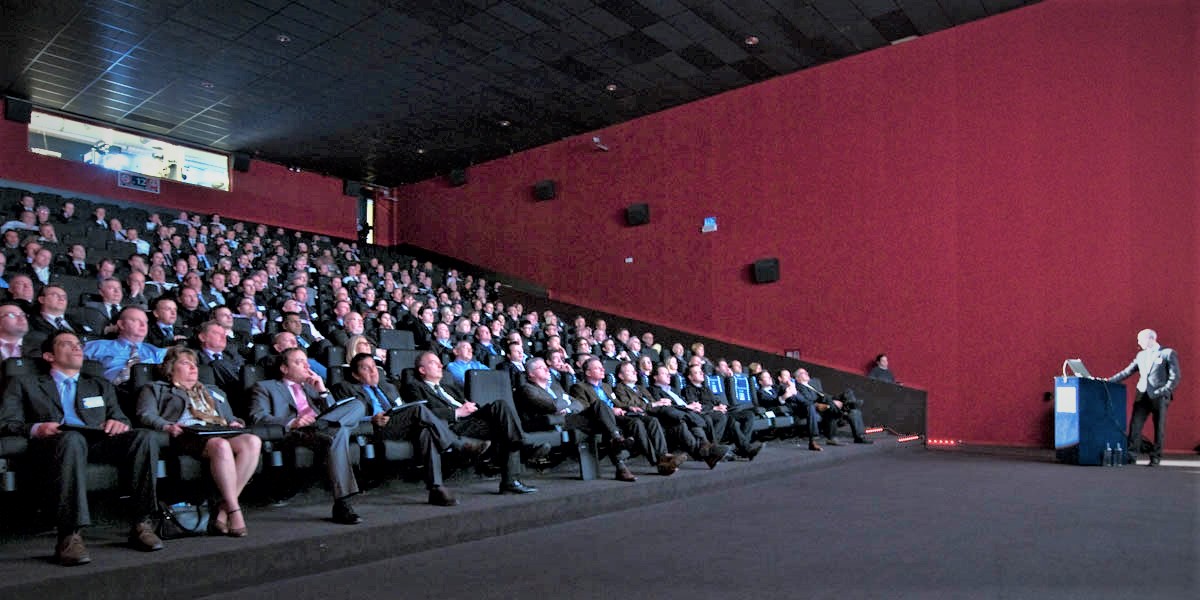
Dr Yannis Kalfoglou
Experiences
35 years of experience and exposure to a multitude of industries including financial services, consumer electronics, consulting, high precision manufacturing, research and development at large FG500 multinationals, SMEs and startups.
Strategic, tactical and hands-on leadership with key technology game changers: AI and blockchain.
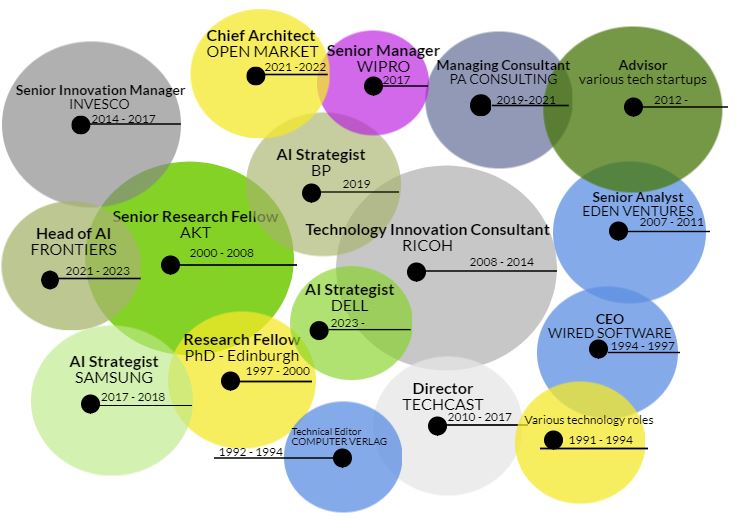