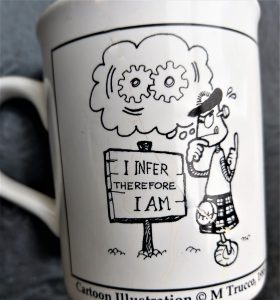
The transformative power of AI: handle with care
Artificial Intelligence (AI) has become of age. Since its very early days, from the Dartmouth Conference in the mid 50s, and the early research labs in Europe and Japan , AI has grown considerably and these days enjoys widespread recognition and awe from practitioners and beneficiaries of AI technology. AI has also gone through periods of lesser excitement, with similar promises which unfortunately didn’t live up to its lofty expectations and resulted in “AI winter” periods. But today, 60 odd years since its foundations as a science and engineering discipline, despite the current hype on AI the prospect of another AI winter is remote, and AI is poised to become the engineering foundation of 21st century technology systems. The kind of autonomous, intelligent, resilient and fault tolerant systems that will serve our coming world of IoT, drones, synthetic-everything and self-driving vehicles.
Regardless of whether we will get to that utopia world sooner than later, we need to focus more resources on work to ensure we fully grasp and understand each evolutionary step of AI before we embark on to the next one. There is no doubt that, eventually, AI can deliver (fragments of) the automated world we used to read and watch in sci-fi movies. But how we get there is crucial.
Commercial push
First, there is an unprecedented boom and commercial interest (AI to become a $50B industry by 2020) for AI applications. Large technology conglomerates drive much of that interest, as the stakes are higher than ever: mastering the current state-of-practice and owning the state-of-the-art for AI gives you a unique competitive advantage over your peers. But the narrative ought to be more open and point not only to the great things that AI can do today, but also all the things that we can’t do (at least not yet)
- Creative tasks (generic problem-solving, intuition, creativity, persuasion)
- Adaptability (situational awareness, interpersonal interactions, emotional self-regulation, anticipation, inhibition)
- Nearly instantaneous learning (AI systems need humongous data sets for training, on the contrary, humans deploy common sense, general knowledge and associative cognition techniques to grasp new concepts and adapt almost instantaneously in new situations)
State of practice
Second, there is the research, engineering and academic practice of AI today: much of the focus and success stories of AI nowadays are in the machine learning (ML) sub-field of AI, with renewed interest in neural networks – NNs (and their recent incarnation, deep learning (DL). Distinctly different from the GOFAI (good old fashion AI) practice, these engineering breakthroughs owe much of their ingenuity to a clever combination of mathematics, statistical sciences and attempts to simulate human neural networks. That connectionism approach to emulate intelligence works well in certain domains but we need to consider the down side when applying these at will:
- Known unknowns: with the current practice of deep neural networks, those that have hundreds of layers like for example the winning NN for the ImageNet image recognition competition had more than 160 layers, it is plausible to arrive at answers that although they appear correct there is no way we can comprehend and trace the answer’s origins. This is a known problem acknowledged even by pioneers in this field. This could lead in situations where AI becomes increasingly difficult to figure out how it achieves its results; not being able to explain why the answer is the way it is or simply unpack the reasoning steps that were used to derive that answer. Progressively trying to understand interactions between NNs is one way of uncovering the reasoning steps, down to the neuron level.
- Right tool, wrong problem: today’s practice of NNs and more specifically DL and in general data science use a plethora of well-known algorithms, techniques, and engineering knowledge from statistics, maths and neuroscience- typically dozens of algorithms are available for any given problem. But choosing the right algorithm for the right problem is not an easy task. Even with the right apparatus in hand, we could still end up misinterpreting our assumptions for the real world and lead to selection bias
- Game for the rich? Undoubtedly, NNs have achieved incredible things over the past 4-5 years, from the Jeopardy TV quiz show to AlphaGo conquering to playing Poker, and beyond. Regardless of the endless possibilities that these engineering feasts open up, we should also note that the AI systems built to achieve all that used vast resources and effort: for example the AlphaGo used 280 GPUs and 1920 CPUs; this is a lot of hardware in absolute terms; both teams at IBM and Google used dozens of the world’s brightest engineers and millions of dollars invested. Progressing AI to the next level it will almost certainly mean make it affordable for everyone, and efficient down to the level of a google search query. We are not anywhere near that yet, but initiatives for democratizing AI can certainly help move us to the right direction.
- Give me (more) data: Current AI practice is data hungry. The more the better. And these data should be, ideally, clean of any noise that could result in contradictions at the training phase. For example, leading ML systems used millions of labelled images for identifying correctly the object at question; in contrast, a young child can use only one image. We need less data hungry and more intelligent, symbolic based input for our state of the art ML systems.
Also need to bring the symbolic AI and connectionism to work together, like the alphaGo use of tree search and other search algorithms in combination with its deep learning network.
Impact
Third, nowadays more than any other part of AI’s history, we need to worry and care about governance and protocols for AI applications. Jobs’ automation, elimination of entire processes and tasks, and even industries are possible with widespread uses of AI. Regardless of the mitigating measures to turn this around and make AI an economic boon for everyone, we need to consider how we regulate AI applications and prepare for its (future) impact:
- Augment, not replace jobs: Automation of manual, repetitive tasks is coming, and is coming fast. And AI is main culprit. But, automating somebody’s job does not equate automating the entire job function and replacing the job holder with an AI driven machine. What we could automate is the repetitive, manual, tedious tasks of the job that occupy a significant portion of someone’s daily job. In recent survey of US occupations these tasks found to be taking up more than 50% of time spent at work
:
All that freed-up and capacity could then be used to do higher order cognitive tasks that we can’t automate and need human judgement. Essentially, we aim to augment jobs, not eliminate them.
- Self-regulate: Government led, industry best practice, legal mandates, and other stipulations could provide guidance on how to bring in and use AI automation, but self-regulation could also work: use of workforce and organisational dynamics to inform best strategies for automation – when, where and how? For example, Aviva, a large UK insurer polled its employees on the impact of robots automating their jobs. This direct approach gives transparency to the process and explores execution paths in a coherent and open manner. Retraining possibilities for workers whose jobs could be automated, include writing the scripts and business logic that robotic process automation (RPA) tools will use – therefore augmenting their jobs to a higher cognitive order, not replacing them; but working alongside their RPA mechanical peers.
- Ethics: the widespread applications areas for AI gives us an interesting puzzle: who’s going to govern AI applications and in what areas? Say, you’re concerned about critical national infrastructure, and AI technology, inadvertently impacts the cyber-defences of a nation, you naturally trying to restrict its uses and reach. But what if the same technology is used to fight back the cyber threats, and identify or cure diseases or used to provide banking services to the billions of unbanked? The decision making of ethics’ committees should be elevated beyond popular opinions from celebrated leaders, business gurus,and luminaries and based on industry, sector and application specific frameworks with input and regulatory oversight built in to ensure alignment with societal values. Dealing with future AI applications is even more challenging as more is yet to come: for example, carefully trained AI systems could predict accurately human right trials, paving the path for a future of AI and human judges working in sync. replace human judges. In these situations we should seek to develop AI systems that move away from the binary nature of their answers and accommodate other paradigms, such as fuzzy systems or good enough answers in the context of open, semantic systems.
Clearly AI is going through a remarkable renaissance. Attention, funding, applications, practitioners, systems and engineering resources were never so many, and concentrated in its 60-odd history. But as AI becomes increasingly relevant to almost every industry and human activity we also need to be aware of the need to regulate and channel its transformative power to truly enhance human lives and experiences.