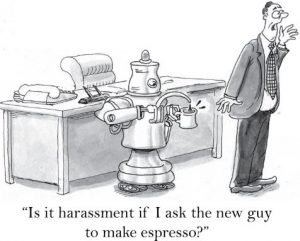
AI watchdogs: do we need them?
The recent advances of Artificial Intelligence (AI) and remarkable progress has caused concern, even alarm to some of the world’s best known luminaries and entrepreneurs. We’ve seen calls in the popular press for watchdogs to keep an eye on uses of AI technology for our own sanity and safety.
Is that a genuine call? Do we really need AI watchdogs? Let me unpack some of the reasoning behind this and put the topic into perspective: why AI might need a watchdog; who will operate these watchdogs and how; and whether it will make any substantial difference to silence the critics.
Watchdogs & Standards
But first, a dictionary style definition of a watchdog: “a person or organization responsible for making certain that companies obey particular standards and do not act illegally”. The key point to bear in mind here is that a watchdog is not just a monitoring and reporting function of some sort, it should have the authority and means to ensure standards are adhered to and to make sure that companies that develop AI do so in a legal manner. I think that is quite tricky to establish now, given the extremely versatile nature of AI and its applications. To understand the enormity of the task, let’s look at a similar, if not overlapping area, that of software engineering standards and practices.
Software engineering is a very mature profession with decades of practice, lessons learnt, and fine tuning the art of writing elegant software that is reliable and safe. For example, international standards are finally available, and incorporate a huge body of knowledge for software engineering (SWEBOK) which describes “generally accepted knowledge about software engineering”, it covers a variety of knowledge areas, and has been developed collaboratively with input from many practitioners and organisations from over 30 countries. Other efforts to educate and promote ethics in the practice of writing correct software emphasize the role of agreed principles which “should influence software engineers to consider broadly who is affected by their work; to examine if they and their colleagues are treating other human beings with due respect; to consider how the public, if reasonably well informed, would view their decisions; to analyze how the least empowered will be affected by their decisions; and to consider whether their acts would be judged worthy of the ideal professional working as a software engineer. In all these judgments concern for the health, safety and welfare of the public is primary”. But these efforts did not appear overnight. Software engineering standards, principles and common practice took decades of development, and trial-error to come up with a relatively condensed version of standards we have today; from over 300 standards from 50 different organisations we had 20 odd years ago.
But, even with carefully designed standards and decades long of acceptable common practice in software engineering we seem that we can’t eliminate the uncomfortable occurrence of the infamous (software) bugs. As everyone who is remotely interested in the safety of software based systems would know, getting it right is not easy: over the years, we have had numerous software disasters, even ones that caused fatalities, loss of property and value, caused wide spread disruption, and so on. And all that due to software bugs that somehow creeped through to the final production code. In most cases, the standards were followed, and to large extent the software system was deemed to be okay. But the point is not to discredit the usefulness of standards: it would have been, arguably, a lot worst without having standards to keep things in check and making sure that the software produced in an acceptable manner and would behave as expected, especially in safety critical systems (from nuclear reactors to autopilots). The point to keep in mind, as we consider following this tried and tested approach for AI systems, is that having standards will not prevent the embarrassing, and sometimes fatal, disasters we aim to avoid.
AI is different
AI also brings to the table a lot of unknowns which make it difficult to even start thinking about establishing a standard in the first place: as some of the most experienced folks in this space advocate, AI verification and validation is not easy. We could encounter issues with the brittleness of AI systems, dependencies on data and configurations which constantly change to improve from past states (a key advantage of machine learning, to constantly learn and improve its current state), we develop AI systems that are non-modular, changing anything could change everything in the system, there are known issues with privacy and security and so on.
But the one thing that appears to be the crux of the problem and concerns a lot of people is the interpretability of AI systems’ outcomes: for example, the well-known industry-backed partnership on AI clearly defines as one of its key tenets:
“ We believe that it is important for the operation of AI systems to be understandable and interpretable by people, for purposes of explaining the technology.”
And they are not the only ones; the UK government’s chief scientist has made similar calls: “Current approaches to liability and negligence are largely untested in this area. Asking, for example, whether an algorithm acted in the same way as a reasonable human professional would have done in the same circumstance assumes that this is an appropriate comparison to make. The algorithm may have been modelled on existing professional practice, so might meet this test by default. In some cases it may not be possible to tell that something has gone wrong, making it difficult for organisations to demonstrate they are not acting negligently or for individuals to seek redress. As the courts build experience in addressing these questions, a body of case law will develop.”
Building that case law knowledge will take time, as AI systems and their use matures and evidence from the field feeds in new data to make us understand better how to regulate AI systems. Current practice highlights that this is not easy: for example, the well-publicized, and unfortunately fatal crashes of a famous AV/EV car manufacturer, puzzle a lot of practitioners and law enforcement agencies: the interim report of one of the fatal crashes reported, points to a human driver error – as the driver did not react on time to prevent the fatal crash – but the role and functionality of the autopilot feature is at the core of this saga: did it malfunction by way of not identifying correctly the object that obstructed the vehicles’ route? It appears that the truck was cutting across the car’s path instead of driving directly in front of it, which the radar is better at detecting, and the camera-based system wasn’t trained to recognize the flat slab of a truck’s side as a threat. But even if it did, does it really matter?
The US transportation secretary voiced a warning that “drivers have a duty to take seriously their obligation to maintain control of a vehicle”. The problem appears to be in the public perception of what an autonomous vehicle can do. Marketing and commercial interests seem to have pushed out the message of what can be done rather than what can’t be done with a semi-autonomous vehicle. But this is changing now, in the light of the recent crashes and interestingly enough, if it was AI (by way of machine vision) and conventional technology (cameras and radar) that let us down, the manufacturer is bring in more AI to alleviate the situation and deliver a more robust and safe semi-autonomous driving experience.
Where do we go from here
So, any rushed initiative to put out some sort of an AI watchdog in response to our fears and misconception of the technology will, most likely, fail to deliver the anticipated results. We’d rather spend more time and effort to make sure we push out the right message about AI, what it can do and most importantly, what it can’t do. AI systems and current practice also needs to mature and reach a state where we have, at the very least, a traceable reasoning log and the system can adequately and in human terms, explain and demonstrate how it reached its decisions. Educating the public, law and policy stakeholders is critical too. As the early encounters with the law enforcement agencies in the AV/EV semi-autonomous vehicles show, there is little understanding of what the technology really does, and where you draw the line in a mixed human-machine environment; how do you identify the culprit in a symbiotic environment where is hard to tell who’s in control: human or machine? Or both?
I sense that what we need more is not a heavy handed, government-led or industry lobby-led watchdog, but commonly agreed practices, principles, protocols and common operating models that will help practitioners to deliver safe AI and beneficiaries to enjoy it. It may well be the case that once we develop a solid body of knowledge about best practices, protocols and models, we set up independent watchdogs, free from commercial influence, to make sure everyone is playing by the rules.